Comment
AI-powered ore sorting: transforming mining efficiency and sustainability
Srinivas Kuppa, chief strategy and product officer of SymphonyAI's Industrial division, explains how AI-powered ore sorting can improve efficiency and sustainability.
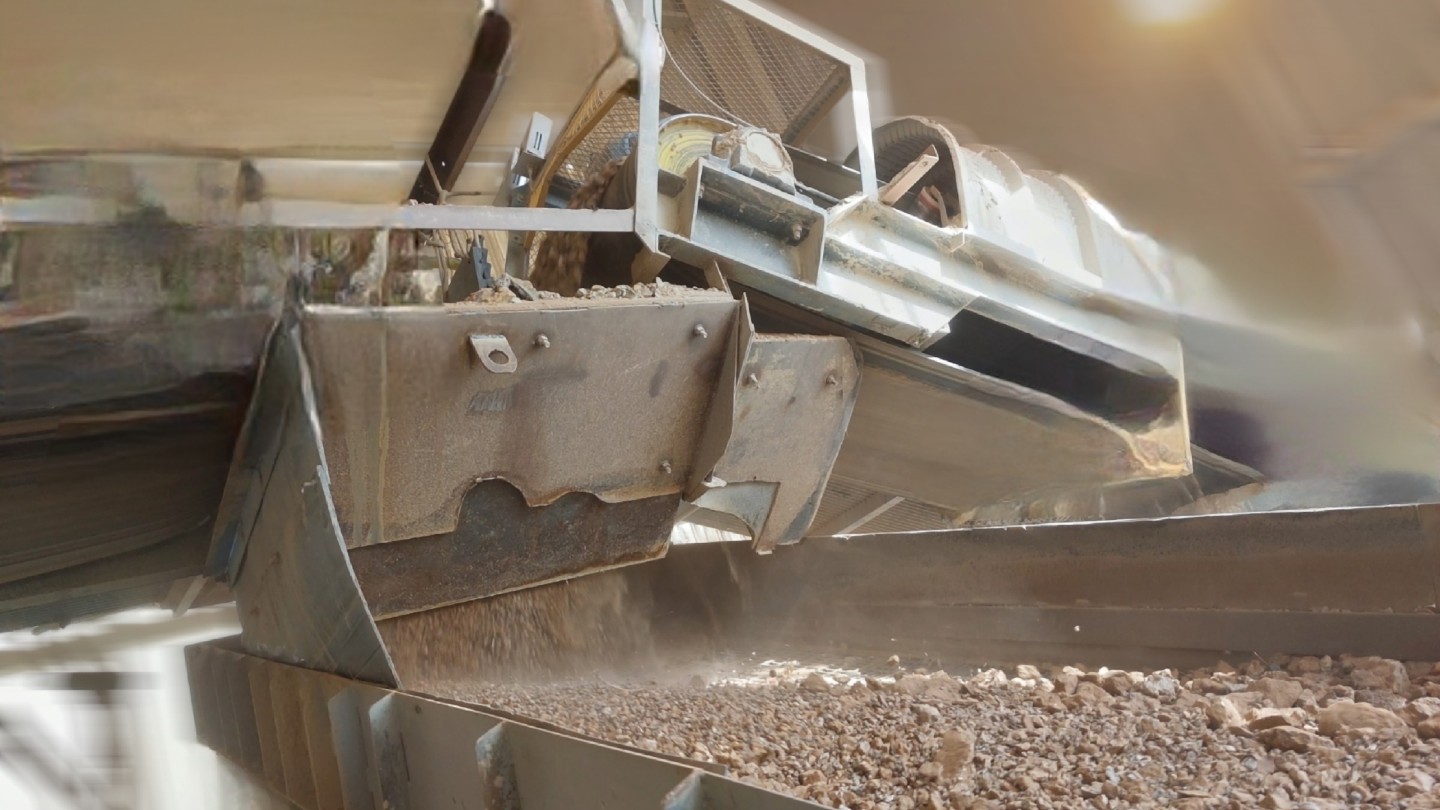
AI technology can support ore sorting in the mining industry. Credit: Gusmary Adriana Briceno / Shutterstock
Environmental concerns, operational risks and plummeting metal prices have led to a steady decline in revenue for major mining operators. The numbers tell a stark tale: the total revenue of the top 40 mining companies plummeted from $943bn in 2022 to $845bn in 2023, with a further drop to $792bn projected for 2024.
Despite these economic challenges, the mining industry has been investing heavily in AI-enabled technologies. This strategic measure has helped them realise a considerable return on investment.
As the mining industry grapples with the dual pressures of cost reduction and improved environmental stewardship, a quiet revolution is underway in ore sorting— a critical process in mining operations.
Here, AI is not just optimising existing processes; it is fundamentally reimagining how we extract value from the earth. This transformation could not have come at a more crucial time, offering a beacon of hope for an industry at a crossroads.
Challenges of low-quality ore transportation
Transporting low-quality ore with high impurities and excessive rock, gravel and dirt reduces mining efficiency. It increases fuel consumption, equipment wear-and-tear, and processing and transportation costs while reducing mineral yield and profitability.
Additionally, environmental impacts due to increased energy use, higher carbon emissions and greater waste generation further strain local ecosystems and resources.
Innovative technologies for ore sorting
To address these challenges, some mining companies are turning to methods of ore sorting, including bulk and particle sorting. Advanced ore sorting techniques such as sensor-based ore sorting (SBOS) offer data-driven advanced sorting techniques.
Various studies indicate that implementing such advanced ore sorting technologies can reduce transportation and processing costs by 20%-30%.
Various studies indicate that implementing such advanced ore sorting technologies can reduce transportation and processing costs by 20%-30%, translating to annual savings of $5m-$10m for a medium-sized mine processing 10,000 tonnes per day. Additionally, improved mineral yield can increase revenue by 5%-10%, potentially adding $25m-$50m annually, significantly boosting profitability while minimising environmental impact.
Furthermore, these technologies can reduce energy consumption, water usage and greenhouse gas emissions, resulting in a substantial decrease in the overall environmental footprint of mining operations.
The evolution of ore sorting
Traditional ore sorting techniques relied on human visual inspection and extensive sample collection and verification processes.
These methods were slow and labour-intensive, often resulting in missed mineral recovery opportunities. Also, these approaches were less scientific and non-repeatable, limiting the ability to standardise and optimise ore sorting across different mining operations.
Over time, advanced techniques such as X-ray transmission, spectroscopy, and electromagnetic sensors improved ore sorting efficiency.
Over time, advanced techniques such as X-ray transmission, spectroscopy, and electromagnetic sensors improved ore sorting efficiency. However, the process remained slow and inconsistent, particularly with varying rock sizes and formations.
These methods were limited by human configuration, lacking the ability to self-learn or adapt, constraining their effectiveness in complex mining environments. The need for more advanced, intelligent and adaptable methods was imminent.
AI and computer vision
An ore sorting technique that uses an innovative combination of AI analytics of sensor (laser and optical) data and advanced AI machine vision analysis of (X-ray, 3D and multispectral) images has emerged to address inefficient ore sorting challenges.
Such advanced AI-powered systems help sort high-grade ore at extraction sites at a massive with no additional demand for on-site human presence.
Generative AI adds an intelligent layer, providing instant insights, summarising complex data patterns, and communicating results in natural language.
High-resolution cameras and multispectral imaging devices capture detailed visual information while sensors collect data on density, chemical composition and other properties. AI algorithms process this combined data stream in near real-time, enabling precise identification and sorting of valuable minerals from waste rock. Generative AI adds an intelligent layer, providing instant insights, summarising complex data patterns, and communicating results in natural language.
The system integrates with IoT [Internet of Things]-enabled equipment for real-time data collection and processing at the extraction site. Since the system is cloud-based, operators can remotely monitor and receive analysis. The use of edge computing devices ensures low-latency decision-making for immediate ore sorting.
This AI-integrated ore sorting solution aims to increase mineral yield by 15%-20%, reduce transportation costs by 25%-30%, and minimise environmental impact through more efficient resource utilisation.
Case studies in AI-powered ore sorting
Some leading mining companies are integrating AI and sensor technologies to enhance ore sorting operations. Freeport-McMoRan has optimised its concentrators with AI models, improving metal recovery and increasing throughput by 10%-15%.
Meanwhile, China's Fankou lead-zinc mine utilises Ganzhou HPY Technology's dual-energy X-ray machines, rejecting over 105,000tpa of waste rock and enriching ore grades from 3% to 12%-14%.
Wolfram Bergbau und Hütten in Austria employs Tomra's XRF sorters to reject 80,000tpa-120,000tpa of waste, enhancing head grade and reducing processing costs. Redwave's XRF-based sensors and other technologies sort various metals, including copper, lithium, and nickel. These advancements highlight the industry's commitment to improving efficiency, reducing costs, and enhancing sustainability through innovative ore sorting solutions.
The future of AI-driven ore sorting
Incorporating advanced ore sorting technologies and real-time data analysis significantly enhances mining efficiency, reduces costs and minimises environmental impact, ultimately driving profitability and sustainability in the industry.
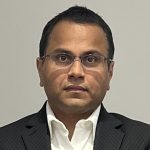
Srinivas Kuppa, chief strategy and product officer at SymphonyAI’s Industrial division
As AI continues to evolve and integrate with other cutting-edge technologies, the mining sector stands poised for a new era of innovation, efficiency, and environmental stewardship.
About the author: Srinivas Kuppa, chief strategy and product officer at SymphonyAI’s Industrial division, has more than 20 years of experience working in AI/ML. He currently focuses on providing business-critical solutions for smart manufacturing, connected asset monitoring and analytics, and mobility using predictive and generative AI.