The matrix below details the areas in AI where mining companies should focus their time and resources. We suggest that mining companies invest in technologies shaded in green, explore the prospect of investing in technologies shaded in yellow, and ignore areas shaded in red.
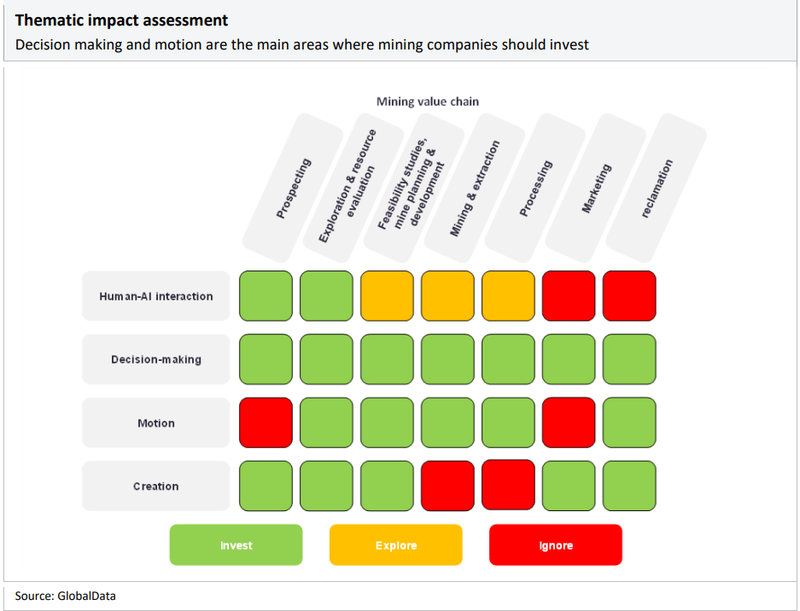
We suggest that mining companies continue or start investing in the motion side of AI as a priority. Other than the marketing and prospecting activities, smart robots and motion control can be used across the whole mining industry. This can be seen already with the introduction of autonomous vehicles and tele-remote machinery. These are especially important for the mines in remote locations, with firms benefitting especially strongly in the mining, extraction, and processing stages.
The creation-side capabilities of AI are also applicable to mining. Mining is not generally associated with generative AI, however, there are clear opportunities where new generative AI technologies can be used across several aspects of mining. It will be especially important for firms to invest in this technology for both marketing and feasibility studies, where it will vastly improve efficiency.
Lastly, the human-AI interaction side seems the least attractive aspect to invest in, although computer vision does relate to how AI is used in mining. It can be used in the first two sections of the value chain to help identify geological areas with rich resource deposits. There are also relevant applications in extraction and processing where computer vision cameras are used to actively monitor the safety of employees.
How AI helps tackle the challenge of safety
Artificial intelligence is going to be imperative to improving safety around mines. Predictive technologies can improve employee protection by providing new real-time insights to avoid dangerous situations and to establish alerts to immediately mitigate risky conditions.
Additionally, AI will allow many of the jobs inside the mines to be replaced as more activities are automated. The most effective way to reduce risks around a hazard is to remove it entirely and automation can enable that. Any underground task is dangerous when performed by a human and the primary benefit of robotics in this area is simple: if a robot can go underground and do a job, humans no longer have to.
Remotely operated drones and land rovers can perform inspection tasks and remotely operated or autonomous rock cutters and borers can perform the excavation and ore extraction. An example of this automation can be found in the automated rock bolting systems. This technology uses machines to virtually eliminate the need for manual labour when installing support bolts into unstable or fractured ground. By using these systems, miners can spend less time in dangerous areas and more time following safety procedures.
Furthermore, automated rock bolting systems have been proven to be much faster and more accurate than manual methods, reducing the risk of underground accidents. Automation also allows mines to monitor the quality of their work better, which leaves the mine’s structural integrity stronger than humans could.
Inspection drones can fly directly into the site without overburdening the structure and continuous cutting vehicles can cut tunnels in regular rectangle shapes, which have better integrity than the tunnels produced by detonation. In June 2022, Newcrest trialled an autonomous inspection ‘SmartHog’ equipped with LiDar, a stereoscopic camera, radar, and LED spotlights. Anglo American developed the Rapid Mine Development System with Atlas Copco (now Epiroc) to excavate low-profile tunnels in hard rock quickly and efficiently.
How AI helps resolve the challenge of productivity
AI has the potential to significantly enhance productivity in the mining industry by addressing various challenges.
AI will be essential in the real-time monitoring of machines and the analysis of data, using machine learning. New mining technology already has real-time monitoring sensors and data gathering capabilities, but the data is at present not being fully utilised. When a piece of machinery is on the verge of failure, it exhibits various signs, such as vibration, overhearing, loss of power, and so on. Machine learning can process and understand this data in real-time, using it to predict when a machine is about to fail and to stop it from operating before it does so, therefore improving productivity. This will eradicate the catastrophic machine failures in the mines, while also reducing repair costs for the mining company.
In a similar way to how automation can improve safety in the mines, it will also improve productivity. Manned machines must stop for shift changes and breaks, but autonomous vehicles boost productivity because they only need to stop for fuelling or maintenance, running as long as you need them.
There’s no risk of drivers getting bored or tired while performing repetitive tasks, so human error is non-existent with these unmanned vehicles. This means the machinery can be working for 24 hours a day at peak performance with no worries of slowing down. As seen below BHP are the leading mining company currently using these autonomous vehicles with 470 trucks now fully autonomous.
How AI helps resolve the challenge of ESG
Mining companies are known for having low ESG scores, often choosing to invest in improving operational efficiency rather than finding methods to reduce their impact on society. This mentality is changing now, however, with the rise in importance of ESG scores to investors and society in general.
For this reason, mining companies might look to AI to help transform their business across all three aspects of ESG, by providing data-driven insights, enabling better monitoring and reporting, and supporting more effective decision-making.
On the environmental side, AI can be used to analyse satellite imagery to monitor deforestation, assess land degradation, and track changes in vegetation cover. This information can be used to identify areas that require conservation measures and to monitor the effectiveness of reforestation and land restoration initiatives. AI systems can identify patterns, predict outcomes, and evaluate the effectiveness of various mitigation strategies.
This can help mining companies optimise their operations to minimise negative environmental effects. AI also has the potential to greatly mitigate and reduce negative environmental effects. Sensors and cameras can be used to monitor mines and gather data, which is then analysed to better understand how waste can be reduced and how to be more energy efficient.
Shyft Inc., a company that integrates leading-edge technological solutions which improve mining production processes, is currently doing just this, using AI, data, and machine learning to forecast energy peaks. AI can analyse data much faster than humans and so better monitor and control the ventilation of mines, greatly increasing energy efficiency and reducing costs in the process.
AI can be used to improve the social impact of mining. For example, AI has been used by companies in Australia as it can help in identifying areas where Indigenous communities are at risk of being affected by mining activities. AI algorithms can also be used to analyse social media and other online platforms to understand the views of local communities on mining activities and identify potential concerns.
Lastly, AI can be used to improve governance and transparency in the mining sector. For example, AI can be used to monitor compliance with regulations and to identify potential violations. Additionally, AI can help in improving supply chain transparency by tracking the origin of raw materials and identifying potential ethical issues.
How AI helps resolve the challenge of cost control
Mining firms are finding it much harder to secure financing for new developments today as compared to 20 years ago, which has led to a crisis of significant underinvestment. This has led companies to seek out novel and often more expensive ways to raise capital including niche funds, high net wealth individuals, royalties, and crowdfunding.
These mining companies are also facing rising operational costs, from inflation, rising labour costs, and geopolitical complications and therefore they are now looking for new technologies to invest in to find cheaper methods of production. Artificial intelligence can be seen as one of the most important technologies to help address these challenges and improve productivity.
One use case for AI is the opportunity of predictive maintenance. AI can be utilised to analyse sensor data from mining equipment and machinery, identifying patterns that indicate potential equipment failures or maintenance needs. By implementing predictive maintenance strategies, mining companies can schedule maintenance activities proactively, reducing unexpected downtime and optimising equipment performance.
This approach minimises costs associated with unscheduled repairs and maximises overall operational efficiency. Reactive maintenance costs enterprises billions each year in lost production. The ultimate goal is not to replace machines or parts too early but service them at the right time.
Similarly, it can be used to optimise resources such as fuel, water, and energy, in mining operations. By analysing data from various sources, including weather conditions, equipment performance, and production targets, AI systems can optimise resource allocation and consumption.
As mentioned previously, artificial intelligence is the technology behind autonomous operations. AI-powered autonomous systems, such as autonomous haul trucks or drilling rigs, can operate with minimal human intervention.
These systems leverage AI algorithms, computer vision, and sensor technologies to navigate and perform tasks efficiently. By replacing or supplementing human operators with autonomous equipment, mining companies can reduce the rapidly increasing labour costs and solve the labour and talent.
How AI helps resolve the challenge of resource development
As all the minerals that companies are mining for are finite, mining firms are constantly looking to find new sources of minerals. Mining companies have started using artificial intelligence to help them identify these mineral reserves. AI can help mining companies analyse large volumes of geological data, satellite imagery, and historical records to identify potential mineral deposits.
It can be used to gain more knowledge and understanding of the terrain and to predict and map it out to a much more precise degree than is possible by human effort alone. Pattern matching and predictive analysis are combined with AI’s ability to analyse large amounts of geological data to reveal the most likely locations of mineral deposits and other resources. Machine learning algorithms can detect patterns, anomalies, and indicators of mineralisation, thereby assisting in more efficient exploration and target selection.
This increase in accuracy helps to reduce the time and money spent on exploration which leads to a much better return on investment. Canadian company GoldSpot Discoveries have been using AI to analyse swathes of data and discover new zones of gold in Newfoundland and Labrador, Canada, proving that companies who employ this new technology are the ones who will lead the industry.
There are also specialist AI vendors in mining discovery such as DroneDeploy, Earth AI, and GoldSpot Discoveries. GoldSpot’s LithoLens uses computer vision on drill core photos to create up-to-date geological logs. Drill Core photos, outcrop images, borehole sensor data, and other existing images are cleaned and optimised to recognise varying geological intervals and specific features such as veins.
GlobalData, the leading provider of industry intelligence, provided the underlying data, research, and analysis used to produce this article.
GlobalData’s Thematic Intelligence uses proprietary data, research, and analysis to provide a forward-looking perspective on the key themes that will shape the future of the world’s largest industries and the organisations within them.