Mining companies are investing in AI to enhance productivity, safety, cost-efficiency, and mineral discovery. The impact of AI in mining is already significant and will only grow in the coming years.
AI enables mining companies to deploy autonomous machinery and use data analytics to enhance operational efficiency and productivity. Autonomous equipment can operate at peak efficiency around the clock, ensuring uninterrupted operations.
Advanced AI algorithms enable predictive maintenance, reducing downtime by preventing accidents before they occur. Predictive maintenance systems can minimize such costs for mining companies while also preventing costly capital expenditure on equipment in cases where assets are irreparable. Advanced AI algorithms also facilitate real-time operational adjustments, further augmenting efficiency.
The mining industry is extremely dangerous. The US Mine Safety and Health Administration reported 42 mining fatalities in 2023. AI plays a crucial role in enhancing safety in mining by automating many dangerous jobs inside mines. The most effective way to reduce risks around a hazard is to remove the hazard entirely; automation can enable that.
AI enables mining companies to use sensors, real-time data, and analytics to understand when changes in factors such as temperature and vibrations can lead to danger. AI-powered wearable sensors can continuously monitor mine workers for signs of drowsiness, fatigue, and physical discomfort, enabling proactive measures to remove workers at heightened risk of accidents.
The matrix below details the areas of AI in which mining companies should focus their time and resources. Mining companies should invest in technologies shaded green, explore the prospect of investing in technologies shaded yellow, and ignore technologies shaded red.
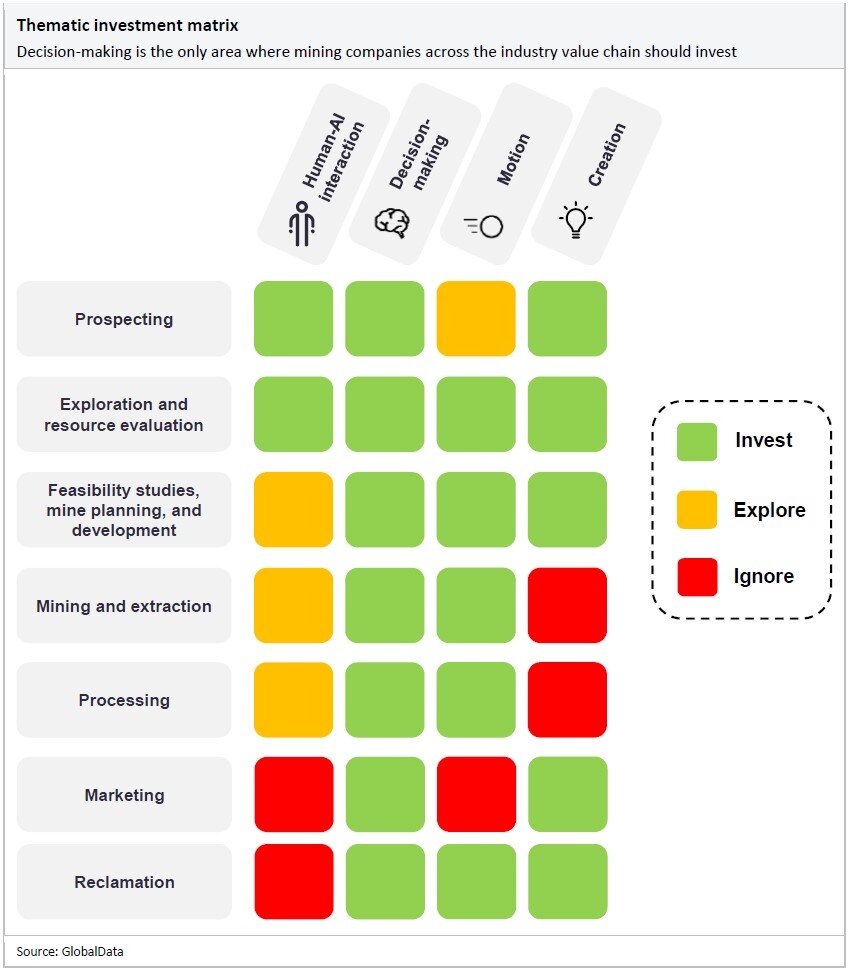
Mining companies must prioritize investment in the motion-side of AI to remain competitive. Aside from prospecting and marketing activities, smart robots and motion control can be used across the mining industry. Autonomous mining equipment, such as autonomous haulage trucks and inspection drones, are already being deployed in mines globally to boost productivity and safety. This technology is particularly crucial for mines located in remote areas, where it delivers significant advantages in the mining, extraction, and processing phases.
AI’s decision-making capabilities are also important for enhancing operational precision and efficiency. By analyzing extensive datasets, AI algorithms help companies make more informed decisions, predict equipment failures, and optimize resource allocation. This leads to significant cost savings and enhanced overall performance.
The creation capabilities of AI are also applicable to mining. Mining is not generally associated with generative AI, but these technologies can support marketing departments by creating personalized content that enhances stakeholder engagement. Generative AI can also support maintenance teams with immediate answers to technical questions, thereby boosting productivity and reducing downtime.
Human-AI interaction is the least attractive aspect of AI for mining companies. However, there are some use cases, such as in extraction and processing, where computer vision cameras can actively monitor the safety of employees.
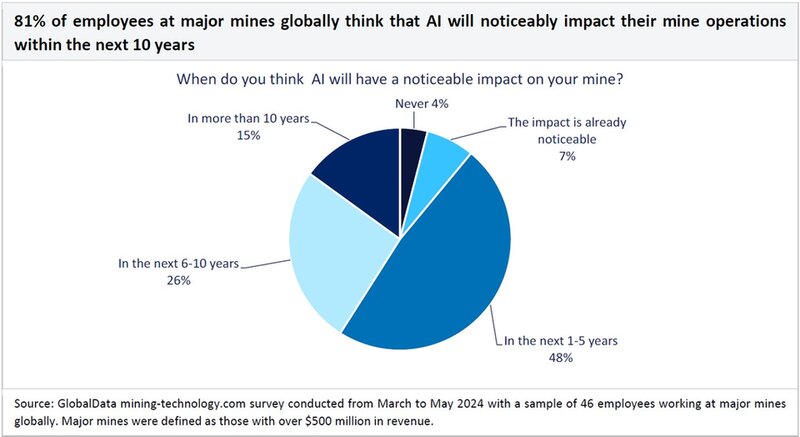
According to a 2024 GlobalData mine-site technology adoption survey, 96% of employees at major mines globally believe AI will have a noticeable impact on their mines. However, 54% of employees identified AI technologies' lack of proven effectiveness as the primary obstacle to investing in AI within the mining sector.
Technology transformation teams within mining companies must showcase the concrete benefits and reliability of AI solutions to address reluctance towards adoption. This will include assuaging employees' fears of job displacement due to AI implementation, a challenging issue that must be carefully navigated.
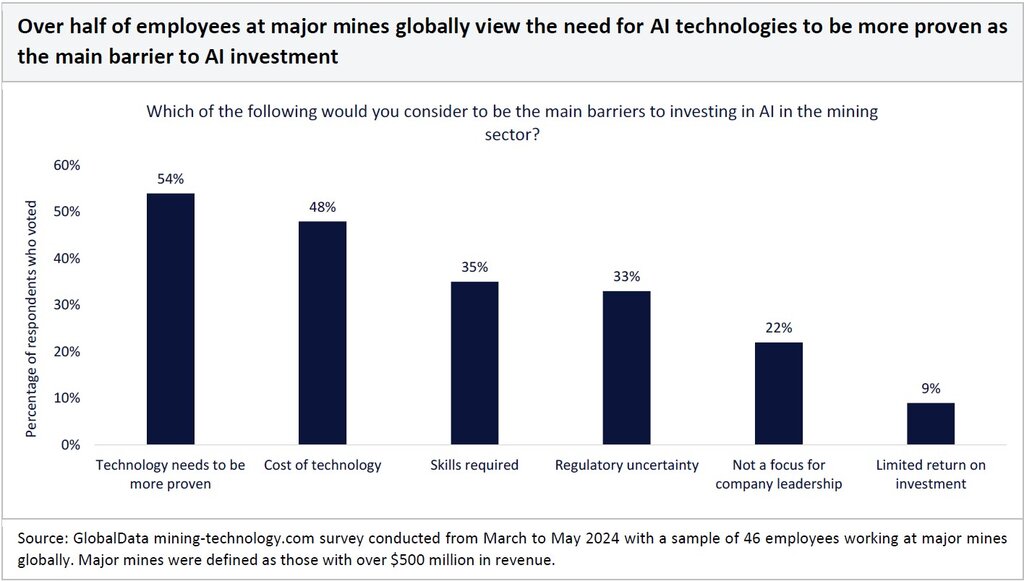
How AI helps tackle the challenge of safety
The mining industry remains extremely dangerous, even with significant reductions in mining fatality rates over the past century and the advent of modern technology. The US Mine Safety and Health Administration reported 42 mining fatalities in 2023. Mining for metals or minerals at great depths poses numerous risks, including exposure to poisonous gases, extreme heat, equipment failure, and geological instabilities that can lead to cave-ins.
Miners are prone to accidents while working in industrial settings involving remote locations, deep mines, pressure variations, and heavy equipment. They are also more likely to develop chronic health conditions. Mining companies must ensure that workers are properly protected on-site by taking steps to avoid accidents and monitoring safety. Accidents can cost lives, devastate communities, repel investors and talent, inhibit production, and lead to the loss of operating licenses, litigation, and insurance claims.
AI plays a crucial role in enhancing safety in mining by automating many dangerous jobs inside mines. The most effective way to reduce risks around a hazard is to remove it entirely, and automation can enable that. Remotely operated drones can perform inspection tasks, while autonomous rock cutters can carry out excavation and ore extraction. Autonomous trucks can also handle loading and haulage, eliminating the risk of accidents caused by human driver fatigue and poor judgment. As of July 2024, GlobaData’s Mining Intelligence Center was tracking 2,080 autonomous haul trucks operating on surface mines worldwide, with these vehicles reducing accidents by 80%, according to a GlobalData survey.
AI is also essential to improving safety through predictive maintenance. AI systems can continuously monitor and analyze operational data to predict potential equipment failures and structural weaknesses, allowing for timely interventions that prevent catastrophic accidents. According to IBM’s technology leader for the EU and NATO, Dirk Claessens, IBM “conducted a study of the coal mining industry in China, where 3,000 people are killed every year. 80% of these deaths were caused by equipment failure. So, there's a clear connection to a safety use case for predictive maintenance."
AI-enhanced equipment performs tasks faster and more accurately than humans, minimizing miners' time in hazardous environments. Automated rock bolting systems are quicker and more precise than manual methods, reducing underground accident risks. Inspection drones can access sites without stressing the structure, while continuous cutting vehicles create uniformly shaped tunnels with better integrity than those made by detonation.
AI-powered wearable sensors can also continuously monitor mine workers for signs of drowsiness, fatigue, and physical discomfort, enabling timely interventions to prevent accidents. The lack of natural light in underground mines can increase fatigue and impair decision-making. Mining tasks can also be physically demanding, and travel to remote locations is exhausting.
A Caterpillar study found that as many as 65% of truck driving accidents in open pit mines are related to fatigue. AI-powered wearable sensors can alert drivers and backroom staff when they are at an increased risk of accidents. BHP Billiton is deploying this technology, having started to use AI-powered drowsiness caps in its Chilean copper mine in 2022 to monitor drivers' brainwaves for signs of fatigue.
How AI helps tackle the challenge of productivity
Mines must ensure that they are increasing productivity by adopting the latest technology. Several factors, including scarcity of minerals and increasing demand, have spurred a shift to more underground mining and remote deposits, leading to longer haul distances, falling ore grades, and rising material and labor costs.
While using AI in predictive maintenance will be essential for improving safety in mining, it also plays a key role in boosting productivity. When a piece of machinery is on the verge of failure, it exhibits various signs, such as increased vibrations, overheating, and loss of power. Machine learning can process and understand this data in real-time, using it to predict when a machine is about to fail and stop it from operating before it does so, improving productivity. A 2024 Siemens report revealed the world’s 500 largest companies lose nearly $1.4 trillion annually due to unplanned downtime, equivalent to 11% of their revenues. Predictive maintenance systems can minimize such costs for mining companies while also preventing costly capital expenditure on equipment in cases where assets are irreparable.
AI also plays an important role in optimizing mining operations. AI algorithms can continuously monitor equipment across various metrics, making real-time adjustments to enhance efficiencies. BHP and Microsoft have partnered to optimize ore processing and grade recovery at Escondida, Chile, the world's largest copper mine. Escondida’s concentrator operators adjust operational variables that affect ore processing and grade recovery by using real-time data from the concentrators in combination with AI-based recommendations from Microsoft’s Azure platform. AI systems can also identify various types of metals more accurately and efficiently than traditional methods, boosting productivity.
Manned machines must stop for shift changes and breaks, but autonomous vehicles only need to stop for fueling or maintenance, running as long as necessary. There is no risk of drivers getting bored or tired while performing repetitive tasks, so human error is nonexistent. This means the machinery can work 24 hours a day at peak performance. As shown in the graphic below, BHP is the leading mining company currently using autonomous vehicles in surface mines worldwide.
While automating mining equipment and vehicles boosts productivity, increasing digitalization in mining operations also raises the risk of cyberattacks. Therefore, mining companies must invest in strong cybersecurity measures to safeguard against crippling attacks.
How AI helps tackle the challenge of resource development
One of the mining industry’s biggest challenges is identifying and accessing viable mineral resources. There is pressure on mining firms to identify realistic new mines. This is made more difficult by an environment of declining ore grades. Locating new mineral deposits or determining the economic feasibility of extracting known resources can be challenging. This is exacerbated by rising development costs and more remote deposits. The exploration and evaluation processes require significant investments in terms of time, money, and technology.
Mining companies have started using AI to help them identify these mineral reserves. AI can help mining companies analyze large volumes of geological data, satellite imagery, and historical records to identify potential mineral deposits. It can be used to gain more knowledge and understanding of the terrain and predict and map it out to a much more precise degree than is possible by human effort alone. Pattern matching and predictive analysis combine with AI’s ability to analyze large amounts of geological data to reveal the most likely locations of mineral deposits and other resources. Machine learning algorithms can detect patterns, anomalies, and indicators of mineralization, thereby assisting in more efficient exploration and target selection. This increase in accuracy helps to reduce the time and money spent on exploration, which leads to a much better return on investment.
Specialist AI vendors in mining discovery include ALS GoldSpot Discoveries, DroneDeploy, and Earth AI. ALS GoldSpot Discoveries’ LithoLens uses computer vision on drill core photos to create up-to-date geological logs. Drill core photos, outcrop images, borehole sensor data, and other existing images are cleaned and optimized to recognize varying geological intervals and specific features such as veins.
How AI helps tackle the challenge of cost control
Mining firms are finding it much harder to secure financing for new developments today than 20 years ago, leading to a crisis of significant underinvestment. This has led companies to seek out novel and often more expensive ways to raise capital, including niche funds, high net wealth individuals, royalties, and crowdfunding. Mining companies also face rising operational costs from inflation, higher labor costs, and geopolitical complications. Therefore, they are turning to technology to find cheaper production methods. AI technologies are among the most important in helping address the challenge of cost control.
As discussed, using AI for predictive maintenance will enhance productivity and safety in the mining industry. However, it is also crucial for effective cost control. By implementing predictive maintenance strategies, mining companies can schedule maintenance activities proactively, reducing unexpected downtime and optimizing equipment performance. This approach minimizes costs associated with unscheduled repairs and maximizes overall operational efficiency. Reactive maintenance costs enterprises billions each year in lost production. The ultimate goal is not to replace machines or parts too early but to service them at the right time.
Similarly, AI can be used to optimize resources such as fuel, water, and energy. AI systems can optimize resource allocation and consumption by analyzing data from various sources, including weather conditions, equipment performance, and production targets.
AI-powered autonomous systems, such as autonomous haul trucks or drilling rigs, also offer mining firms significant cost savings. Despite high initial investments, these systems reduce labor costs and mitigate talent shortages by performing tasks with minimal human intervention through advanced algorithms, computer vision, and sensor technologies.
How AI helps tackle the challenge of ESG
Environmental, social, and governance (ESG) issues increasingly influence how business is conducted globally. Stakeholders demand greater transparency and action on a full spectrum of ESG issues. Companies must perform well in all 12 aspects of GlobalData’s ESG framework; being a laggard in any one will damage brand reputation and, ultimately, profits.
The mining and metals industry accounts for approximately 4% to 7% of worldwide greenhouse gas emissions. By its very nature, mining depletes natural resources and significantly impacts surrounding ecosystems. However, metals and minerals will be pivotal in the global transition to a greener future. As scrutiny of ESG issues intensifies, mining companies will use AI to make their operations more environmentally and socially sustainable.
On the environmental side, AI can be used to analyze satellite imagery to monitor deforestation, assess land degradation, and track changes in vegetation cover. This information can be used to identify areas requiring conservation measures and track the effectiveness of reforestation and land restoration initiatives. AI systems can identify patterns, predict outcomes, and evaluate the effectiveness of various mitigation strategies. This can help mining companies optimize their operations to minimize negative environmental effects.
AI also has the potential to mitigate and reduce negative environmental effects. Sensors and cameras can be used to monitor mines and gather data, which is then analyzed to understand how waste can be reduced and how to be more energy efficient. AI can analyze data much faster than humans and so better monitor and control the ventilation of mines, greatly increasing energy efficiency and reducing costs in the process.
AI can also improve the social impact of mining. For example, AI algorithms can analyze interviews, social media, and other online platforms for local community feedback and sentiment on mining activities, enabling mining companies to understand and address their needs and concerns. This can foster more sustainable development and prevent public relations disasters.
Lastly, AI can be used to improve governance and transparency in the mining sector. For example, AI can help improve supply chain transparency by tracking the origin of raw materials, which can enable more accurate and complete Scope 3 emissions reporting and identify potential ethical issues.
GlobalData, the leading provider of industry intelligence, provided the underlying data, research, and analysis used to produce this article.
GlobalData’s Thematic Intelligence uses proprietary data, research, and analysis to provide a forward-looking perspective on the key themes that will shape the future of the world’s largest industries and the organisations within them.