The Best Technologies for Predicting Earthquakes
Scroll down to read the article
On 26 February, a 7.5 magnitude earthquake ripped through Papua New Guinea, claiming the lives of 160 people. At the time of writing, it remains the deadliest quake to strike this year.
Several mines that dot the pacific island – one of the world’s most resource-rich countries – were among the areas hit. Ok Tedi Mining, which operates a large gold mine in the country’s Enga province, reported significant infrastructural damage due to a landslide triggered by the quake.
The Porgera gold mine, also situated in Enga, sustained damage to its primary power generating facility; however, its Chinese operator, Zijin Mining Group Company, reported minimal damage beyond this, with no employees injured during the initial quake or subsequent aftershocks. And in the immediate aftermath, the mine was still able to operate on back-up power.
Things could have been a lot worse for Papua New Guinea’s miners, but this incident highlights the risks of exploration in earthquake-prone regions. Furthermore, it poses the question: what difference can seismic monitoring technology make in the monitoring and prediction of earthquakes to help miners prepare for such events?
Tests on longwall coal mines
One technology that could make a difference is microseismic monitoring, which is able to record, analyse and interpret smaller quakes in the event of geological materials fracturing and failing. This can happen around underground excavations, such as longwall coal mines. The analysis of three component microseismic data can provide the location of the failure, as well as detailed information about the fracturing process’s mechanisms and parameters.
The applied & environmental geophysics research group at Keele University has applied the technique to the monitoring and predictions of outbursts in longwall coal mines in both the UK and Australia.
“The nature of caving behaviour in the vicinity of longwall extraction can be critical to the financial success of a colliery,” reads an extract on the group’s official website. “Face support, interaction effects, optimum pillar design, subsidence at surface and control of water all depend critically on the distribution of fractures and stress induced by mining. Until now, there has been no method by which these effects could be observed.”
Recently, the research group collaborated with UK company IMC Geophysics to create a new solution based on cementing seismic detectors, known as geophones, into a smaller number of in-mine boreholes to record simulated micro-earthquakes during mining operations.
According to the group, careful analysis of these recorded signals produced a map of the caving activity, “leading to increased understanding of all aspects of caving”. This included the ability to observe the creation of caving features, highlight areas of abnormal caving, deduce optimal panel widths, and discover falling pillars.
Careful analysis of these recorded signals produced a map of the caving activity
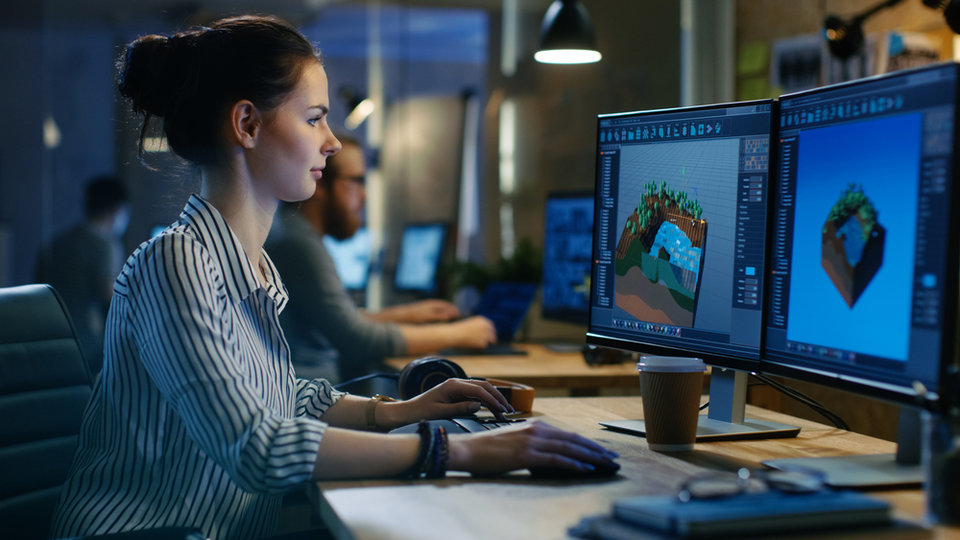
Monitoring historical seismic data helps build intelligence about hot spot locations.
The major challenge is to obtain accuracy in prediction with considerable time complexity
Is
machine-learning the answer?Artificial intelligence may also have a part to play. In 2016, a data mining competition organised by the University of Warsaw was held in Poland, where data scientists from across the world were invited to come up with new algorithms to predict earthquakes. More than 200 participants were provided with two datasets comprised of seismic activity records from 24 operational Polish coal mines. One was for training, consisting of 133,151 observations and the other for testing, made up of 3,860 observations.
With the goal of predicting seismic events up to eight hours in advance, prediction models were judged in their accuracy in respect to the area under ROC curve (AUC) metric – the standard classification analysis used to determine best prediction models. The eventual winner was Michał Tadeusiak, a big data science specialist with California-headquartered deepsense.io, which achieved an AUC of 0.939.
The results prompt a further question when it comes to AI as, with such large datasets, what constitutes a significant observation over a 24-hour period? According to Tadeusiak, there are 13 general features, which include the ID of the working site, as well as the overall energies derived from “bumps, tremors, distressing blasts and total seismic energy”.
In India, researchers at the Sinhgad Institute of Technology and Science in Narhe, Pune, have also been examining how machine-based learning could help workers foresee and prepare for earthquakes in a coal mine setting.
In a paper from last year, the group proposed a prediction system based on datasets of attributes such as seismic energy, total energy, seismic bumps and shift, while suggesting various algorithms – such as neural networks and feature engineering – could also be used for training.
“The major challenge is to obtain accuracy in prediction with considerable time complexity,” the paper states. “We can say that the mining-induced seismicity can be a mini model depicting earthquakes. Thus we can say that the proposed prediction system can be used for further earthquake prediction as well. The proposed software framework gives a user-friendly interface for coal miners that would either alert them or notify about dangers on basis of predictor system.”
Finally, it seems that for now, while microseismic monitoring is a tool that has been deployed in the mining industry for some time, as means of ensuring greater operational safety, its main function for miners remains prevention rather than prediction.
We are seeing a huge interest and traction on how to use technology to optimise