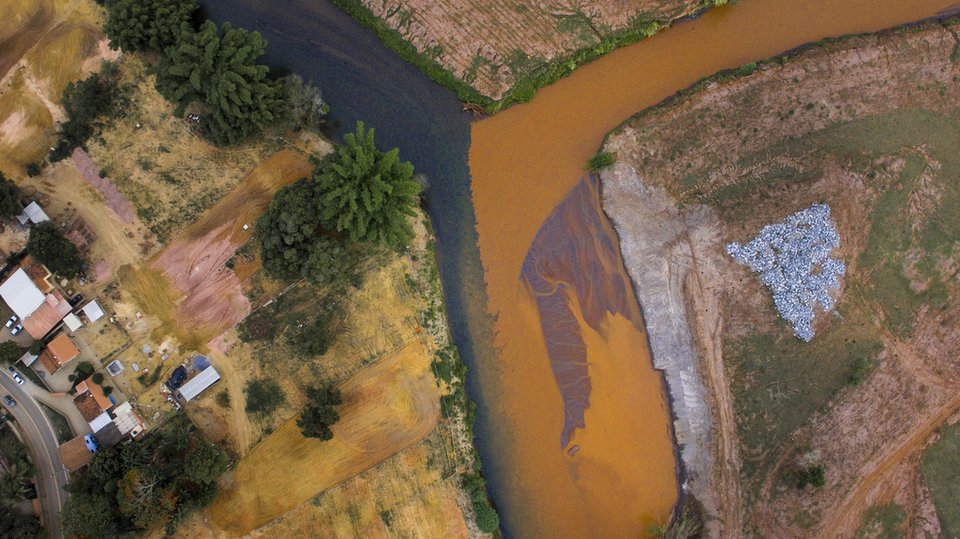
AI
How AI can revolutionise the monitoring of tailings facilities
Tailing dam failures continue to be a real and present danger for mining operations. Zachary Skidmore investigates the potential of autonomous drones and AI in enhancing the monitoring of these facilities.
A
rtificial intelligence (AI), when matured, will revolutionise the way that heavy industry operates. Within the mining industry, it is applicable in a range of services, from geo-mapping in the planning stage to monitoring and evaluating the mine site during operations.
Still, autonomous technology is probably the area that has the most considerable potential to disrupt the current practices of the mining industry. Autonomous drones are already a feature on several mine sites, operating in a monitoring and evaluation capacity.
This offers a great opportunity to alleviate many of the risks that employees take as part of their daily routines. The most prominent of these is the inspection of tailings dams, which are particularly significant as there have been 37 major tailings dam failures in the last decade alone, with these events becoming more frequent.
The use of autonomous drones to collect consistent and reliable data on the tailing facilities could mitigate many of the concerns associated with sporadic in-person monitoring. That data can then be disseminated into a platform through AI and machine learning to provide accurate data insights in real time, allowing the relevant stakeholders to ensure these facilities’ structural integrity, safety, and reliability, while remaining in compliance with regulations.
Tailings and inspection processes
Tailings dams are built to last in perpetuity; however, in the context of long-lived infrastructure, the rate of failure of tailings dams is high. Many tailings dams suffer from inadequate design regulations, as well as less rigorous construction and supervision methods than for standard water-retaining dams.
Currently, 90% of reported tailings failures occur within active mines. The distribution of tailings dam incidents by country indicates that 74% of the cases come from a small number of countries and regions: the US (39%), Europe (18%), Chile (12%), and the Philippines (5%). However, the lack of accurate information from developing countries and a credible database means that many tailings failures go unreported.
Disasters to the scale of the Brumadinho dam failure in January 2019, which led to the deaths of 270 and around 12 million cubic metres of tailings being discharged into the local environment, are few and far between. However, the rate of failures has increased in recent years, with around half of the significant tailings dam failures in the last 70 years occurring between 1990 and 2010.
Water will determine our survival as a people beginning now and into the future, especially with climate change.
At present, most tailings are inspected regarding their potential hazard classification, whereby more at-risk dams are inspected more regularly. All require inspection by competent geotechnical engineers. However, most of these inspections occur infrequently, meaning mine operations rarely have real-time data on the status of the dam.
A significant challenge in this process is that tailings dam failures are seldom the result of a single action but rather a failure of the entire system. The most common cause is overtopping, the result of overfilling at a rate that the dam is not designed to tolerate, often after heavy downpours. This makes tailings facilities very unstable as failures can occur at a rapid rate, making it next to impossible for the mine staff to respond.
AI and its application
AI-driven drone technology has the potential to revolutionise the process of monitoring and evaluating these facilities. Using digital technologies, mining operations can enable the continual surveillance of a global network of tailings facilities using mobile, connected sensors; satellite data; and video feeds from drones or fixed points.
With this collected data, companies can deploy advanced analytic platforms to generate key business insights, indicate risk levels, and provide recommendations for mitigating actions. The relevant stakeholders can then view the visualisations to enable effective and timely decision-making processes for dam maintenance.
Detailed data from tailings dams, when continuously gathered, analysed, and modelled, holds great potential for improving safety in tailings dam management.
Lyzandra Boshoff, consulting principal engineering geologist at mining and metals consultancy SRK, told Mining Technology: "Detailed data from tailings dams, when continuously gathered, analysed, and modelled, holds great potential for improving safety in tailings dam management. The data is particularly useful when it can give a real-time picture of changing conditions in tailings dams."
Several organisations have sprung up offering AI-driven autonomous drone solutions applicable to mine operations. Percepto, for instance, offers a range of autonomous drone solutions, using machine learning and AI to feed the data gathered by their drones into a wider platform that disseminates the data to relevant stakeholders on any mobile device, enabling effective action before escalating into more serious problems.
Percepto CEO Dor Abuhasira, in an interview with Mining Technology, spoke of his platform's applicability to the monitoring of tailings facilities: "The client can program an autonomous drone to inspect them twice a day, providing data on the status of the materials inside, how heavy they are, and what actions can be taken to prevent catastrophes."
Benefits and weaknesses
The use of AI-driven drones in inspecting tailings facilities has a range of benefits, including the ability to generate significant amounts of data with much less effort, which can be digitally communicated in real time. A limitation of the traditional system was that the data might not reflect the prevailing conditions in the tailings dam when the information reaches the engineer for analysis.
Additionally, there is the immediate and obvious advantage of machines being able to work around the clock without experiencing fatigue and being able to access areas and environments that may be too dangerous for humans.
Dr Penny Stewart, managing director of PETRA Data Science, in considering the effectiveness of AI monitoring of tailings dams, said: "There are different types of data that you get from monitoring tailings dams, including hydraulic pressures, displacement data, and visual imaging from satellites.
"I expect data platforms capable of near real-time integration of all monitoring data will enable more complete and complex monitoring of tailings dams, including potentially machine learning."
Therefore, AI could revolutionise our understanding of tailings facilities, allowing for constant and up-to-date data, allowing for greater management of the facilities and, in turn, reduced risk.
Tailings dams can fail very suddenly, and with little warning, particularly if liquefaction of the tailings is triggered.
However, Stewart also tempered this optimism, arguing, "I don't know if this would be enough to accurately predict or prevent a failure. Tailings dams can fail very suddenly, and with little warning, particularly if liquefaction of the tailings is triggered."
To combat this, autonomous drones would have to be in constant operation to ensure that tailings don't suddenly fail. For large, diversified mining companies such as Rio Tinto, this is not an issue. However, in developing countries, the risk is that this technology will be too expensive to be deployed.
Despite this, mining companies should act now to leverage and invest in these technologies towards high levels of deployment throughout global mine operations. Doing so would make business-critical strides toward zero harm.
// Main image: The Gualaxo do Norte river, polluted with mining tailings mud, meets the clean Carmo river in Barra longa, 80km from the epicentre of the Samacro disaster, 1 November 2016. Credit: Gustavo Basso / Shutterstock.com
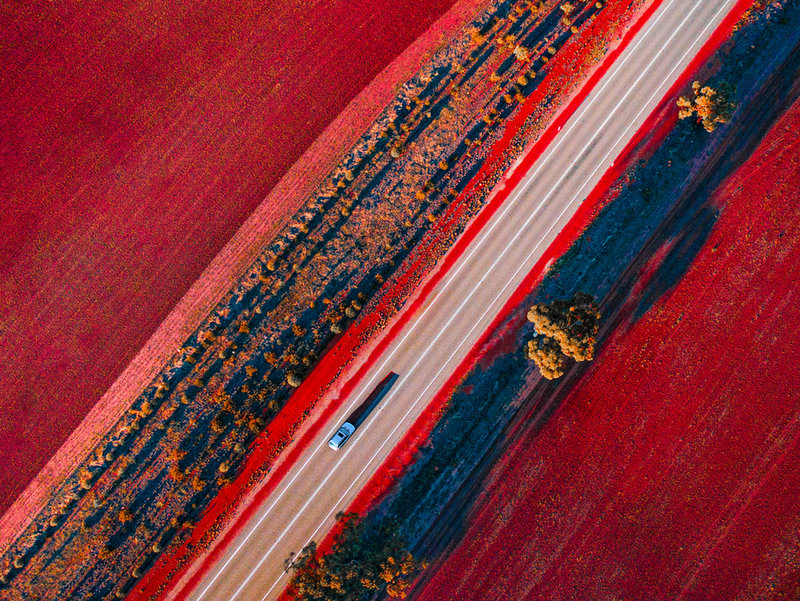
// 3D System Model and Completed Installation. Credit: Deimos